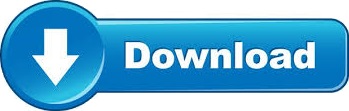
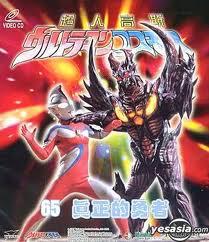
Select from 11 non-spatial covariance types, including first-order ante-dependence, heterogeneous, and first-order autoregressive. Model means, variances and covariances in data that display correlation and non-constant variability, such as students nested within classrooms or consumers nested within families.įormulate dozens of models, including split-plot design, multi-level models with fixed-effects covariance, and randomized complete blocks design. Linear mixed models/hierarchical linear models (HLM) Provide a unifying framework that includes classical linear models with normally distributed dependent variables, logistic and probit models for binary data, and loglinear models for count data, as well as various other nonstandard regression-type models.Īpply many useful general statistical models including ordinal regression, Tweedie regression, Poisson regression, Gamma regression and negative binomial regression Use linear mixed models for greater accuracy when predicting nonlinear outcomes, such as what a customer is likely to buy, by taking into account hierarchical and nested data structures.įormulate dozens of models, including split-plot design, multi-level models with fixed-effects covariance and randomized complete blocks design. Mix and match categorical and continuous predictors to build models, choosing from many model-building possibilities. Use flexible design and contrast options to estimate means and variances and to test and predict means.
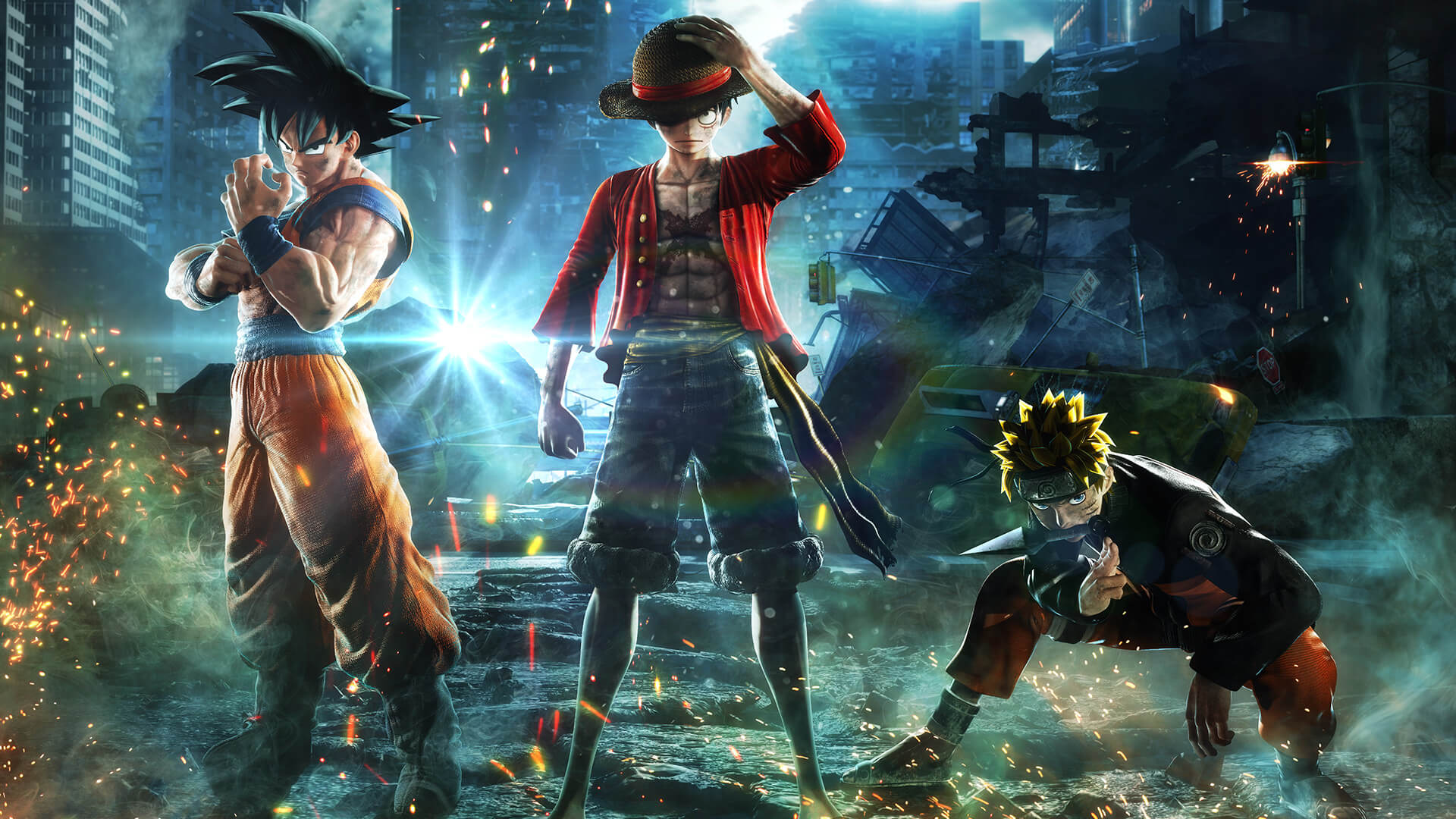
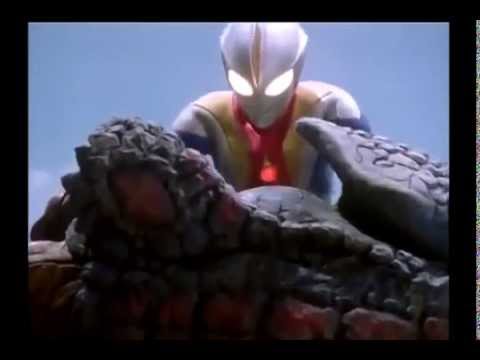
Models include linear regression, analysis of variance (ANOVA), analysis of covariance (ANCOVA), multivariate analysis of variance (MANOVA) and multivariate analysis of covariance (MANCOVA). Survival analysis procedures for examining lifetime or duration data.ĭescribe the relationship between a dependent variable and a set of independent variables. Generalized linear mixed models (GLMM) for use with hierarchical data and a wide range of outcomes, including ordinal values. Generalized estimating equations (GEE) procedures that extend generalized linear models to accommodate correlated longitudinal data and clustered data. Linear mixed models, also known as hierarchical linear models (HLM), which expands the general linear models used in the GLM procedure so that you can analyze data that exhibit correlation and non-constant variability. Generalized linear models (GENLIN) including widely used statistical models, such as linear regression for normally distributed responses, logistic models for binary data and loglinear models for count data.

General linear models (GLM) and mixed models procedures. SPSS Advanced Statistics provides the following capabilities: These sophisticated analytical techniques are frequently applied to gain deeper insights from data used in disciplines such as medical research, manufacturing, pharmaceuticals and market research. IBM SPSS Advanced Statistics provides univariate and multivariate modeling techniques to help users reach the most accurate conclusions when working with data describing complex relationships. Powerful modeling techniques for analyzing complex relationships
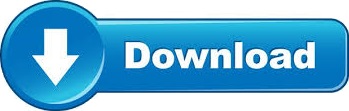